This article first appeared in the Q3 issue of GlobalTrading.
With George Molina, Head of EME Trading, and Kevin Ngan, Quantitative Trader, Franklin Templeton
What is the state of play for using data and quantitative models in emerging markets equity trading? How has this evolved?

The industry has been adapting to automation progressively in the last few years within emerging markets, and the use of algo products such as sell-side algo suites and algo wheels has increased dramatically. The rise of unconstrained algos has created vast volumes of data and more opportunities to leverage data science in trading.
Traditionally, strategies are executed using a trader’s extensive trading experience and market knowledge. As data continues to play a more prominent role, statistical analysis is gaining influence on the modern trading desk. As a desk’s culture slowly gravitates towards a more data-driven and scientific approach, its strategies are constantly challenged and supported by adequate back-testing and hypothesis testing. As a result, buy-side firms have started to seek additional quantitative talents, most often a hybrid between a traditional trader and a quantitative researcher, who can draw actionable insights from millions of noisy data points and maintain a set of quantitative models. On the systems side, there has been advancement in third-party technology, particularly in EMS [execution management system] and TCA [transaction cost analysis] data APIs [application programming interfaces], where it helps to significantly boost research efficiency and the capability to deploy quantitative models in production.
How does low touch in EM equity trading differ from doing the same in developed markets equity trading? What are the unique challenges in EM?
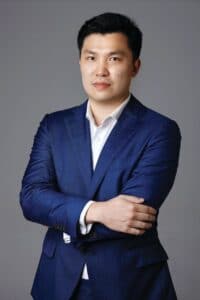
Fundamentally, DM and EM have different areas of interests in terms of execution alpha. DM focuses more on alternative venue analysis and child order routing, and venue toxicity has a greater influence in realized market impact cost. While there are only a handful of dark pools available in EM, the diversity in market microstructure is a more significant factor in post-trade analysis, as each country has its own market characteristics and liquidity profile. One common pitfall is to have a single blanket execution strategy for a whole region, or a series of independent strategies for each country and benchmark; in both cases, a typical buy-side firm will quickly run into a data sampling problem and fail to obtain meaningful results even with years of execution data. Hence, knowing how to reduce dimensions while maintaining statistical significance with enough data points is one of the crucial questions that should be answered with an algo wheel setup.
Another interesting challenge within EM is how far automation can go before sacrificing execution performance. At present, humans are still the center of trading in the region, and algos tend to underperform manual trading in bigger ADV bands, especially in markets where blocks contribute to a big proportion of available liquidity. Ultimately, the sweet spot on the automation spectrum depends on the flow characteristics of the firm, and it is important to maintain excellent and consistent execution qualities.
Does Franklin Templeton use data and quant models in EM equity trading as a differentiator / competitive advantage vis a vis other institutional buy sides?
Rather than viewing data and quant models as a standalone tool, our desk seeks to integrate them into the trading process as much as possible. Data itself is only one part of the equation; a full cycle involves experiments design, data collection, data cleaning, statistical inference/machine learning, model development, and live production. Although the process could be highly quantitative, choosing the correct objective function is a work of art and hugely relies on market expertise, especially for high-touch models where traditional TCA is not the only metric to evaluate routing destinations.
This framework encourages idea generation across the whole team, and allows traders to challenge existing methodologies and fine-tune any parameters. As a result, the team is better equipped to react to market inefficiencies and deliver best execution and client service. The desk has also been constantly engaging with sell side and vendors in leveraging their quantitative resources. It is important to keep an open mind and understand the latest developments, in order to test and apply any relevant ideas into our workflow.
As the industry continues to evolve with an ever more complicated regulatory landscape and higher expectations, a culture of innovation and embracing technological change is vital to drive the success of the buy-side trading desk.
Where is EM equity trading on the spectrum of high touch to low touch? Is it moving towards low touch? Are you seeing a convergence of high touch and low touch?
The share of algorithmic trading in Asia is catching up with Europe and the U.S. At Franklin Templeton, the percentage of algo flow has doubled in the last two years in Asia. Since the introduction of the algo wheel, execution quants are able to design scientific experiments to identify the optimal parameters and conduct broker reviews through statistical inference; this provides a level of confidence among traders and thus selectively moves some flow from high touch to low touch.
Nonetheless, low touch is by no means a substitute of high touch. Each has their pros and cons, especially in Asia. Although the advancement of data and technology will further increase the share of algo trading in the region, there is a cap on how far this can go. This cap will depend on the type of flow and the expected market impact cost. For example, the IOI ecosystem offers sizable liquidity; combined with sensible analysis and scoring, the realized high-touch market impact cost will outperform that of low touch, particularly during times of heightened volatility and spread regimes, as was seen in March 2020.
Overall, high touch is slowly converging toward low touch; equilibrium will be reached when buy-side firms figure out their best order profiling.
What is the future of artificial intelligence and automation in EM?
Going forward, there will be more attention towards the integration of data and high-touch trading. For every small yet crucial manual routing decision, a machine learning model is able to look at historical data and immediately provide suggestions to traders. This could be IOI destinations, broker selection, crossing size and frequencies, etc.
As a traditional asset manager, the human element in trading will still play an important role in Franklin Templeton’s future; with best execution for our clients in mind, the human overlay has proven to be an excellent complement to quantitative models.