By Emanuele Tomeo, Chief Technology Officer at Mosaic Smart Data
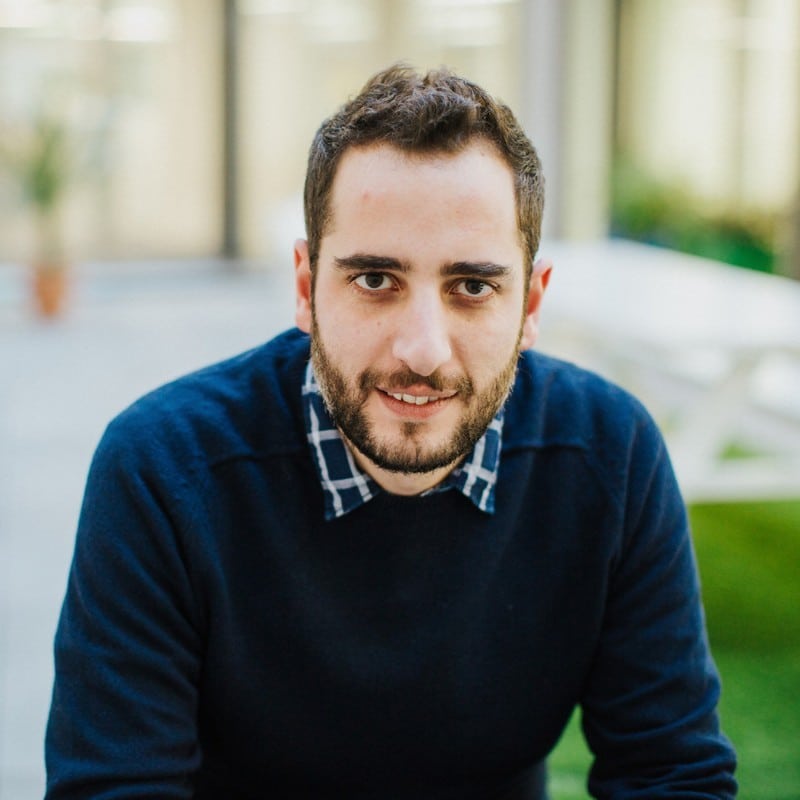
2023 has been a whirlwind of a year for ChatGPT and other Large Language Models (LLMs). These AI platforms have hit the headlines consistently since the year began, for better and for worse. But cutting through the hype, is this technology ready for prime time in global financial markets?
Warnings about uncontrolled development of AI are picking up pace across the industry. Most notably, the ‘Godfather of AI’, Geoffrey Hinton quit Google in May and warned others about the dangers of AI and misinformation, and ChatGPT was recently banned in Italy over privacy concerns.
ChatGPT parent company OpenAI’s mission statement is to ensure that AI benefits all of humanity and to advance it safely. While many have marvelled at the programme’s capabilities from drafting detailed essays in seconds, to writing computer code and job applications, LLMs can at times be deeply flawed and can’t always be trusted – as even the founders of this tech have admitted.
But what potential does this technology hold for banks operating in capital markets, and what risks and drawbacks does it have in its current form?
The ability of models like ChatGPT to process and understand large amounts of data, combined with their advanced language capabilities, opens a wide range of interesting possibilities. Forbes this year, listed ten use cases for ChatGPT in the banking industry. But if we look under the hood, the technology has a number of issues in its current form that prevent it from delivering true value to banks that are looking to gain a comprehensive view of their data and extract actionable insights from it.
1. The data compromise risk
Data is a bank’s most valuable asset, and as such they guard it fiercely. Any risk of a data compromise that could reveal sensitive information to their competitors will see them running for the hills.
ChatGPT’s suspension in Italy over data privacy concerns will have a been a major warning sign for many banks. The inherent manner in which the platform handles users’ data is highly problematic for the financial services industry where confidentiality is key. It relies on the ability to aggregate all the data that users input and use it to continuously train the model’s ability to provide accurate information outputs.
In the world of capital markets, data analytics platforms have strict confidentiality agreements with each of their bank customers that prevent this kind of pooling of data across institutions.
As such, banks require platforms that are able to aggregate all transaction data from across an organisation as well as external sources, normalise it, and apply advanced AI and machine learning tools – all in a secure environment where data confidentiality is key. This enables banks to extract actionable insight without having to risk their data landing in the wrong hands.
2. The challenge of knowing what to ask
When it comes to niche capital markets such as FICC, a careful combination of AI technology and human expertise is required to ensure the output is of use to salespeople and traders.
Banks should look to platforms that have been purpose-built by FICC market experts and which are able to recommend actions that a salesperson or trader might not have even thought to ask about. This enables banks to suggest appropriate opportunities to clients at exactly the right time.
For example, if a client always trades at 2pm on a Thursday, the platform will alert the bank at 1pm to get in touch with the client. Or if a client does their index rebalancing on the 27th of every month, the platform will tell the bank on 26th of every month that client is about to become very active. The result for the bank is increased loyalty and greater share of mind amongst clients.
With ChatGPT on the other hand, you need to know exactly what to ask it to get the output you desire. And this is a skill in itself: what, and when, should you ask, when you can ask anything?
3. Non-deterministic output
Consistency of information is very important in financial markets – banks need to know they are providing credible, accurate and consistent recommendations and advice to their clients.
This is contradictory to ChatGPT, which is constantly learning, and so the answer to the same question can be different each time you ask it. The platform is also being increasingly critiqued for spitting out false information that can propagate so-called ‘fake news’ sites.
A lot of the data in the capital markets field is structured tabular data where other generative AI technologies, like Variational Inference-based Neural Networks and Bayesian Networks, are gaining more adoption. LLMs like ChatGPT are trained to produce an answer that makes sense, rather than be strictly correct. Their main strength is working on unstructured data, as they have been trained on sentences and sequences of text, and they do not work well with large bodies of tabular data or solve advanced numerical optimisation problems
While there are examples of ChatGPT assisting banks in minor transaction activities, flagging suspicious transactions, and identifying potential fraud, on the whole its non-deterministic approach is a high-risk factor for banks. Interestingly, JP Morgan has recently banned workers from using the tool for work-related tasks.
4. Lack of model explainability
Model explainability is increasingly important in the field of data analytics. Banks want to know exactly why they are being given certain intelligence, and how the models underlying AI technology are working.
As a result, their workforce is becoming more fluent in generating and calibrating models to meet their specific needs – and vendors are increasingly working in collaboration with them, rather than the typical vendor-customer model.
ChatGPT, on the other hand, aggregates a huge amount of information and generates an output, but it doesn’t tell you why it has generated the output. Even Google’s CEO Sundar Pichai has admitted that we still don’t fully understand how LLMs work. This can also present a number of compliance challenges, with regulators often requiring banks to be able to explain why they made a certain decision.
Building the foundations for the future
There’s no doubt that this is an exciting time for AI and its capabilities. LLMs have surprised even their creators with their unexpected talents. But for it to be truly useful, this technology must be tailored to the nuances of capital markets and combined with specialised human knowledge.
The Economist summed it up recently when it stated: “Artificial intelligence poses risks, but also offers extraordinary opportunities. Balancing the two means treading carefully. A measured approach today can provide the foundations on which further rules can be added in future. But the time to start building those foundations is now.”