A quick search in Google shows more than 10,000 results for quantamental despite the fact that it is not an official word. The reason, of course, is that traditional active management has been underperforming passive management, particularly when fees are accounted for, and people are therefore looking for new approaches. Quantitative managers, on the other hand, have done well versus their benchmarks in many cases and both Big Data and Artificial Intelligence (AI) generate the type of marketing buzz that buy side salespeople love.
At the same time, for some investors, there is still a stigma attached to fully quantitative asset management. Those fearful investors equate the description of quantitative manager with market neutral hedge funds exclusively, or believe that they rely solely on technical, price momentum strategies using so-called black box algorithms. There are also investors, described in anearlier piece, that have substantial concerns about technology failures or machines running amok. The concept of integrating human judgment with the best techniques of quantitative managers for generating or capturing alpha is, therefore, very appealing. Thus, quantamental was born and is gaining strength as a trend in the financial industry.
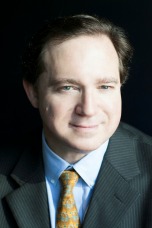
The reality, however, is that there are many misconceptions about quantitative management, how such managers trade and pick stocks and what quantitative techniques can achieve. To start, lets debunk a few myths commonly held to describe quantitative managers:
1)Quantitative managers are exclusively long / short market neutral or global macro funds.While both of those types of funds CAN be a quantitative fund, both long/short or global market funds could also be run traditionally. More important, almost every type of fund run by institutional asset managers can be quantitative, since the main characteristic of quantitative funds is that their opinions on the future performance of assets are expressed numerically and related to a benchmark. A quantitative long only fund, for example, would be overweight stocks which they believe will outperform and either underweight or hold no shares of stocks which they expect to underperform. Such a fund would also likely hold positions of stocks in proportion to their index benchmark weight whenever they did not have an opinion on its expected performance.
2)Quantitative managers exclusively rely on technical price driven formulas, rather than embracing traditional valuation techniques.In fact, there are quantitative approaches to virtually all asset manager sources of alpha. These include a myriad of approaches using Big Data and AI to predict cash flows, industry growth rates, input costs or any other variable considered by traditional managers. In addition, quantitative managers can use data sources that are derived from human sources. Surveys of expected price moves from traders and salespeople on Wall Street can be used to replace phone calls that provide trading color to asset managers, and analyst opinions aggregated by vendors can be used instead of reading the highlights of research reports individually.
3)Quantitative managers operate exclusively based on data analysis without human judgment.In the opinion of many (including yours truly), there are still situations where nuances can be uncovered by human analysts to generate alpha. It is possible for analysts or portfolio managers to add value directly, even in a quantitative fund. The difference, however, is that in a quantitative fund, portfolio managers and analysts would be expected to predict the amount of outperformance or underperformance they expect for each stock instead of determining their desired position sizes themselves. This approach facilitates both a direct, empirical assessment of the accuracy of portfolio managers and analysts and, more importantly, allows quantitative funds to optimize the size of positions in their portfolios relative to the combination of predictions, risks and costs.
4)Quantitative management requires enormous expenditures in terms of data, PhD quants and technology.This one is less myth and more of a synthetic barrier to entry described by the first generation of quantitative managers and others. The first firms did need to invest heavily in all these aspects of infrastructure. Today, however, there are many providers of important components of the quantitative trading process, including data acquisition, data analysis platforms, portfolio construction, order and execution management systems and trading analytic systems. It is still important to have access to data, quantitative talent and technology, but the cost of each component is decreasing due to new providers entering the industry and providing services at a lower cost. The major exceptions to the trend of reducing cost are recruiting, which is increasingly competitive, accessing new (or perhaps even big) data that differentiates the alpha produced by the strategy, and the building of trading systems. Trading systems keep getting more expensive due to escalating market data and co-location costs, but that is a subject for a different day.
Now that I have described what quantitative managersare not, lets describe what their key defining characteristicsare. The best way to do so is to explain the three main areas of difference between fully quantitative asset managers and old school traditional managers: their approach to asset selection (alpha), how they construct portfolios (beta) and how they trade (transaction costs).
Alpha: There are actually two differences between fully quantitative managers and traditional managers with respect to choosing what assets to invest in: the prediction methodology and the method in which predictions are expressed. The prediction methodology itself is typically the most discussed aspect, with many vendors (including theResearch Signalsgroup at IHS Markit) providing tools such as stock screeners, in-depth factor analysis of individual assets or sectors and back-testing engines. The range of these tools, including our own data-feeds, commentaries and papers, is vast, and can help managers conduct research. The end product of such research is to identify undervalued or overvalued companies, sectors or geographies, or the identification of factors that can be used to create portfolios expected to outperform. That said, all managers select assets which they believe will outperform, whether quantitative or not, so another key difference is the method in which predictions are expressed.
Quantitative asset managers express all opinions on individual assets in terms of either outperformance or correlation to a benchmark or both. Such predictions are expressed as actual numbers at a point in time, often with a specific duration. For example, a quant manager may well predict a specific percentage outperformance for a particular stock for the next day, month or year, with the number relative to a broad-based index, a sector sub-index or style index such as value or growth. Traditional managers can express their opinions in many ways, but typically analysts revert either to a buy, sell, hold status or simply express their opinions as orders sent to their trading desk or as target positions to a portfolio construction (risk) group. As a practical matter, one method of being quantitative is to ensure that all assets in a manger’s investible universe are in one of the following groups:
Outperform– Positive alpha is predicted and the manager holds a position larger than the weight of the stock in the benchmark. The amount of the overweight should be proportional to predicted alpha constrained by the excess factor risk (industry, style or other) as well as by the trading cost of acquiring and later liquidating the asset.
Neutral– No opinion or conviction regarding the relative performance of the asset, in which case the asset should be held in the portfolio in the precise weight of the benchmark.
Underperform– Negative alpha is predicted, in which case the asset should be weighted below the benchmark weight or not at all. While a long only manager may not actually short stock, it is important to grasp the concept that not owning a stock that is part of a benchmark is essentially a synthetic short position of that security. This is due to the fact that if that stock outperforms the benchmark, the manager will suffer from negative returns by comparison, but if the stock indeed underperforms, the manager will have positive relative returns. Considering that most equity managers are evaluated compared to index benchmarks, it is clear that even long only managers should be evaluated based on both their negative and positive predictions.
Outperform,but not in benchmark– The manager believes the asset will outperform the benchmark, but the stock itself is not currently in the benchmark index being managed against. The reason that this is a separate category is that such assets hold more risk for the manager, since the default neutral weight is zero, while assets in the benchmark should be held at index weight as a default. That said, human analysts often uncover value in more obscure stocks that might have less data for a machine to evaluate than those in the benchmark. In this case, such securities could be an important source of alpha.
Beta:In the context of this note, beta refers to the movement of the benchmark index or perhaps movement in individual sectors of the benchmark index. Bets on beta movements are relatively common with traditional managers, and can also be part of the strategy of quantitative managers. Traditional managers could end up with beta bets from multiple causes, including, but not limited to, significant cash positions (which are essentially bets against the benchmark index), concentrated positions in stocks with higher or lower individual correlation to the market or sector, aggregate sector weightings different than the benchmark or explicit predictions by the portfolio manager that particular sectors will over or under perform. The difference between such active manager bets and quantitative managers is that the quants always seek to categorize beta risks precisely, measure them as well as their contribution to returns and manage them. Quantitative managers will either utilize predictive models to take beta bets or employ risk models to minimize those bets. In either case, quantitative managers leverage these models within the framework of a portfolio optimizer in order to analyze the portfolios deviation from an optimal target and generate trade lists to move in the optimal direction.
Transaction Costs:Quantitative managers tightly couple the trading process with the stock selection and portfolio construction process. In practice, this means two things. First, pre-trade cost estimates are an input to the optimization process, and second, all orders and trades are measured compared to that pre-trade benchmark. I have already discussed these issues at length in past posts, so will not reprise them here. Read my article onWaters Technologyfor an explanation of why pre-trade cost estimates should be integrated into the investment process and my tworecentpiecesto develop an understanding of the basis for how costs should be measured.
In conclusion, all managers that are not fully quantitative could adopt some or all of the techniques of quantitative managers to improve their investment returns. Becoming quantamental does not require that a manager change their identity, eliminate human judgment or start chasing price momentum. It does, however, require that they introduce more discipline into their investment process by measuring each component and increasing the integration between them. There are many data analysis tools available to fundamental managers to help them create predictions, express them mathematically and do a better job at portfolio construction. Utilizing these techniques and process enhancements to augment their own experience and judgment could potentially improve the performance of active managers that choose the quantamental path.
David Weisberger is Managing Director, Trading Services at Markit