Data coverage, timeliness, and quality issues with historical data was cited as the top challenge in the investment research industry, with nearly two-fifths (37%) of respondents selecting this option, according to a research from Bloomberg.
This was followed by normalizing and wrangling data from multiple data providers (26%), and identifying which datasets to evaluate and research (15%).

Angana Jacob, Global Head of Research Data, Bloomberg Enterprise Data, said these findings are reflective of what they’re seeing in the industry and through many conversations with their investment research and quant clients.
“A main theme that comes through is the challenge clients have with wrangling with and normalizing traditional research and alternative datasets given their sheer size and fast-changing nature,” she told Traders Magazine.
Additionally, she said in order to derive insights or backtest trading strategies, clients have to map and integrate the datasets into existing models and systems.
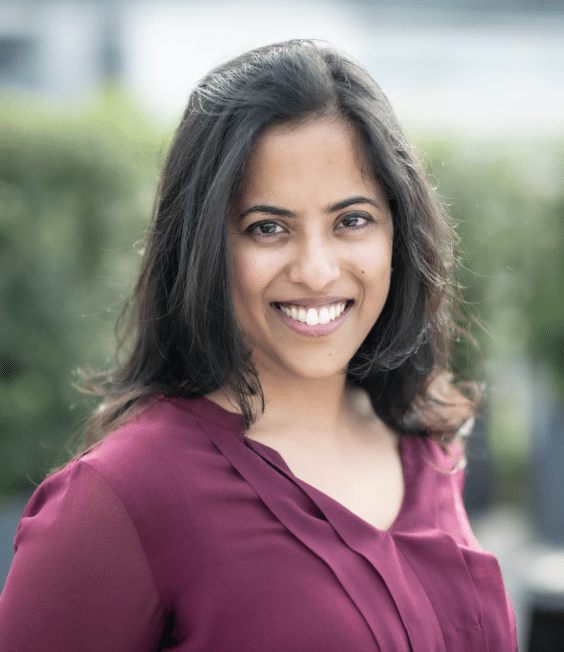
“Harnessing the value and potential alpha of a dataset can only happen after these foundational blocks are painstakingly stitched together, and this requires a big lift from quants, developers, data scientists and data engineers,” she commented.
Bloomberg’s Investment Research Data Trends Survey found that 72% of respondents could evaluate only three or fewer datasets at a time, despite the need from quants and research teams to continually harness more alpha-generating data in today’s data deluge.
The findings also show that the typical time it takes to evaluate a single dataset is one month or longer for more than half of respondents (65%).
According to Jacob, the desire to constantly test and incorporate new datasets is strong but, in line with the findings, typically clients can only evaluate a relatively low number at one time (three or fewer at a time for most respondents of this survey).
Additionally, more than half of respondents typically take one month or longer to evaluate a single dataset, she added.
“To gain a competitive edge in different markets, investors continuously need granular, timely, interconnected data with deep history, and it’s unsurprising that this remains the biggest research data challenge to our survey respondents,” she stressed.
More than six in ten (62%) of respondents prefer their research data to be made available in the cloud.
According to Jacob, the investing landscape has had rapid technology changes and the possibilities for quant investing and alpha generation today are completely different to what existed 5 years ago.
As an example, she said: a few years back, intraday systematic strategies required significant hardware investment and time to backtest and deploy, whereas now with scalable cloud infrastructure, strategies can be faster while markedly more powerful, incorporating more data.
“In today’s environment, customers need seamless access to market information and the ability to handle increasingly vast volumes of data for computationally-intensive investment processes such as backtesting, signal generation, optimizing portfolios, scenario analysis, hypothesis testing and so on,” she said.
“Quantitative analysis and systematic strategies are generally on the cutting edge of technology and we are seeing that with their cloud adoption,” she said.
“Cloud is a critical enabler for providing the scale, elasticity and cost efficiency to run increasingly complex investment research and trading processes,” she added.
Additionally, Jacob said that clients’ integration of Machine Learning and AI into their investment process is well-supported by cloud capabilities, enabling rapid prototyping of new trading strategies and faster simulations with unprecedented volumes of financial and non-financial data.
“Cloud platforms provide the necessary infrastructure spanning cost-effective storage, specialized hardware and elastic computational resources for intensive calculations,” she said.
According to the findings, 50% of respondents reported they currently manage the data centrally with proprietary solutions versus outsourcing to third party providers (8%), with more than six in ten (62%) of respondents preferring their research data to be made available in the cloud. Notably, 35% of respondents also would like their data to be made available via more traditional access methods such as REST API, On premise and SFTP, indicating they prefer flexibility in the choice of data delivery channels.
Jacob commented that typically, quantitative and systematic clients prefer more control and customizability over their investment research and execution process, and therefore would prefer to not outsource their entire tech stack -hence what we see in the survey results.
“However in recent years, we do see greater interest and comfort from these clients in outsourcing vertical slices of their research-to-production lifecycle to specialized providers,” she said.
“Managing the entire tech stack in-house can often detract from the primary goal of generating alpha and outsourcing could bring benefits of reduced operational burden, lower total costs and domain-specific expertise,” she added.