Algo Wheels are not a new concept. Initially designed as a workflow improvement for buy-side traders, they have become much more sophisticated over the years. “I think it’s a tremendous workflow help and assistance for the buy-side. It’s been adopted fairly well from across the buy-side, but the adoption has taken a long time,” commented Mehmet Kinak, Global Head of Equity Trading at T. Rowe Price.
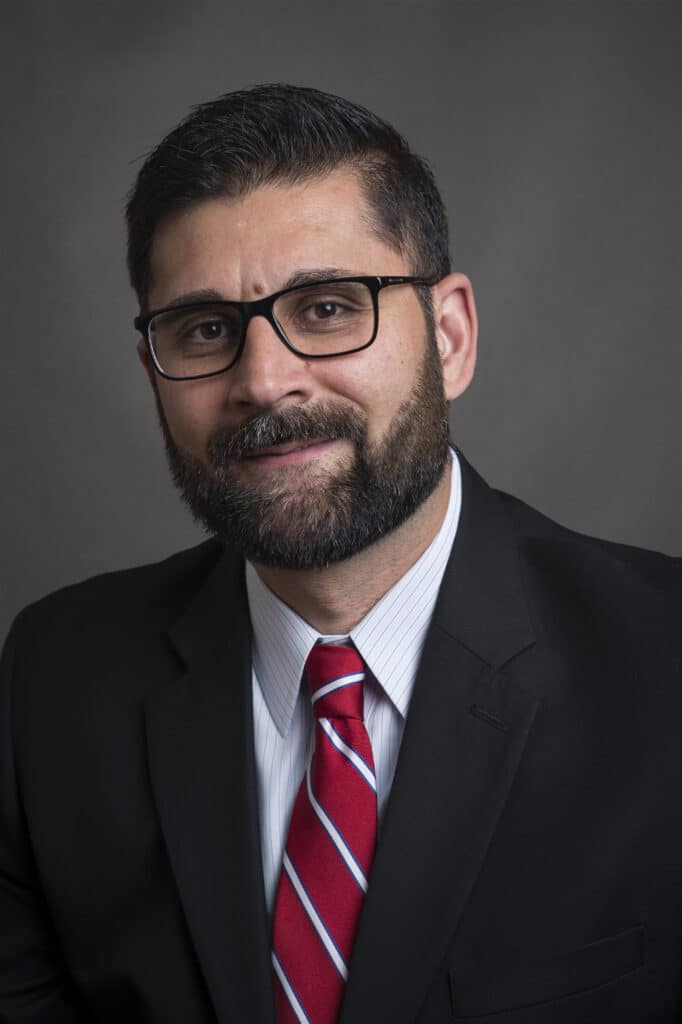
Baltimore-based T. Rowe Price Group, a global investment management firm with $1.51 trillion in assets under management as of February 29, 2024, deployed its first wheel in 2009, Kinak said, adding that he knows some buy-side firms that are still deploying wheels for the first time. “It’s been about a 10 year plus adoption, but one that I think the industry has really benefited from”.
“What you saw early on were very large firms with a lot of different order flow trying to incorporate wheels because it helped from an automation perspective getting a lot of smaller orders off their desks and to algorithmic execution venues,” Kinak explained.
“If I give a human 1000 orders to split evenly, they’re going be close, they’ll do a pretty good job. But I know that a machine will do it without bias, it’ll do it more accurately,” he stressed.
“Initially, it was very basic workflow enhancement – use algorithms to split orders up, so that we can collect and analyze data,” he added.
Kinak argued that wheels have gotten far more sophisticated and part of that has to do with the amount of data that can be incorporated into wheels – data, as in the types of orders that you have, specifically the characteristics of those orders, data around how well different vendors are performing different strategies, even data that captures market dynamics.
“Modern wheels can incorporate a lot of different data points,” he said.
“They have become sophisticated enough and intelligent enough that we can say that we’re executing orders in an optimized fashion,” he added.
“It’s not only a workflow improvement anymore, it has also improved execution quality,” he stressed.
Kinak added that at T.Rowe Price they are using wheels in a multi-factor way: “I could argue that execution quality has improved by use of wheels. And because of that, implicit execution costs have come down,” he said.
From an execution quality perspective, Kinak said, where best execution improves “it’s almost like a circular loop of information”.
“When we use these wheels, they feed back information into our analytics system, where it says, ‘you’ve spread 1000 trades to five different vendors, 200 trades a piece’. Then we can look at the execution quality of those vendors very easily and say, ‘Vendor A seems to perform very well executing small cap securities, whereas Vendor B does very well executing liquid securities’,” Kinak explained.
“Then we can adjust and calibrate the wheel accordingly. It’s a circular loop of information where, as we do more trades, more data gets fed into our analytic system, and we can analyze that data to determine what the best approach would be, either minimizing or maximizing who we’re sending trades to, introducing a brand new vendor, or even a brand new wheel,” he added.
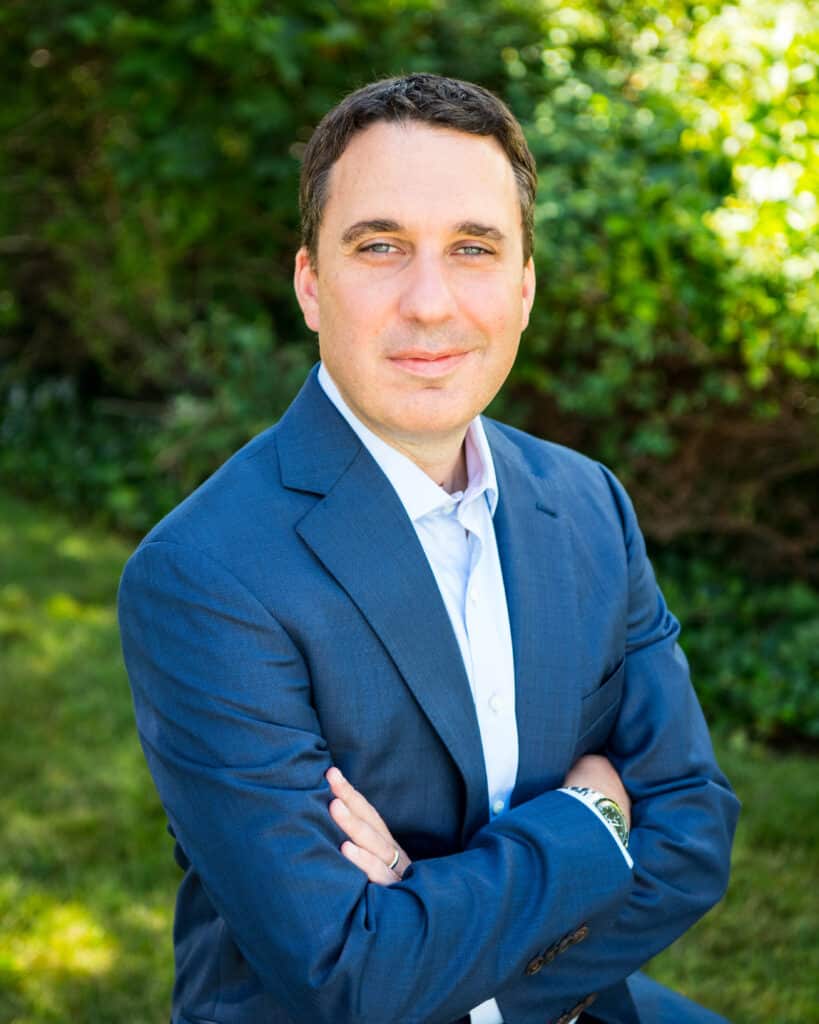
Michael Beattie, Head of Product Strategy, Charles River Development (CRD), said that they have seen different types of wheels being employed. In addition to asset managers, CRD’s clients include asset owners, hedge funds, and insurance companies. “We see automation show up across our entire client base,” Beattie said.
He noted that there are three main types of wheels, all of which are probability based – a random selection/equal weighted type wheel, a custom weighted wheel, and a more advanced wheel that imports external data to drive selections. “From there you can develop a lot more complexity with wheels as you look at different iterations of those three core wheel types and target basis options.”
The most complex clients with $500 Billion AUM and above, with very complex rules, and a large amount of order flow use a combination of three core pillars that envelop wheel usage: classification, order flow management, and automation, he said.
“As you go down the trajectory to firms that don’t have as many orders throughout the day, you see that usage become less,” he stressed.
Vidya Guruju, Director, Product Management at Charles River Development, added: “Global firms prefer to have regional wheels, one per location, and have the desks operate their individual wheels.”
He said that asset managers are quite comfortable with wheels: “There are still firms who are on the fence, but broker wheel usage is becoming more of a norm these days than an exception.”
Benefits, risks and future of algo wheels
The primary benefits of using a wheel are bias removal, best execution, and broker agnostic allocation, according to Guruju.
When asked if algo wheels help reduce trading costs, Guruju said that “it’s more of a side effect or a side advantage than the main one”.
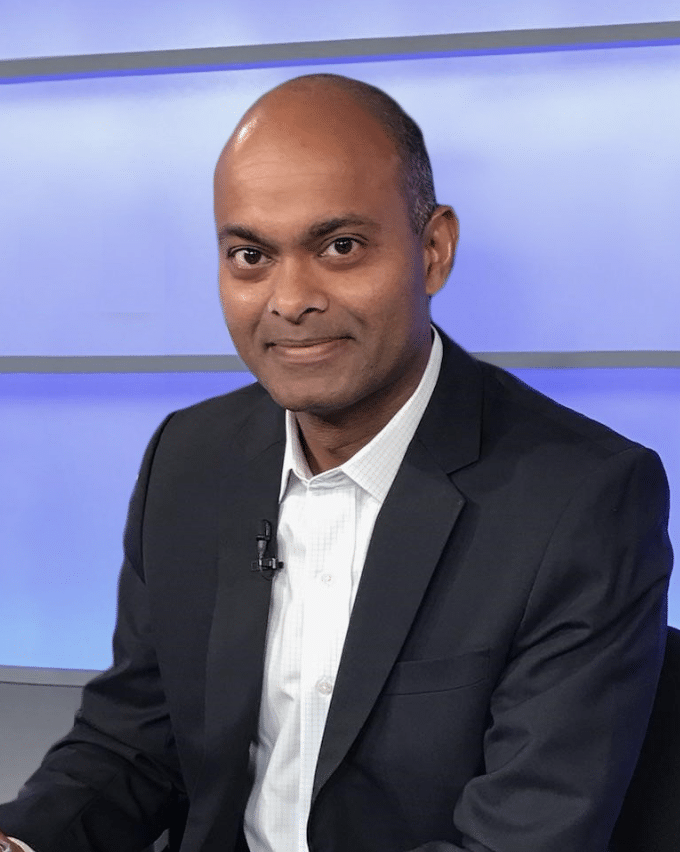
“The fact that you’re able to route similar orders to the same broker reduces those explicit and implicit costs in a way because your market is not moving against you, or you’re not competing with yourself,” he said.
According to Beattie, the wheel helps to dictate best execution to a certain extent: “If you’re managing commissions, there’s a benefit to that. If you’re managing execution quality, there’s a benefit to that as well.”
Kinak added: “I could argue that wheels are actually helping improve execution quality.”
He thinks it’s difficult, even if you’re a smaller firm, to not use them, because if wheels are improving execution quality, you’re almost required to find out if they’re going to be beneficial for you to reduce your implicit transaction costs.
“I think those firms that had ignored them from a workflow enhancement are now saying, ‘okay, I might have to look at this, because it might actually be an improvement to my overall execution quality’,” Kinak said.
“Frankly, I don’t see the downside of having a wheel, the only caution I would put out there is that you need a lot of data to achieve the enhancements to execution quality,” he said.
He said if a smaller firm that doesn’t trade that frequently, adopts a wheel, it might not help them from a workflow perspective because they don’t have a lot of trades to automate: “And if it’s not helping them with execution decisions, because they don’t have a big sample set to utilize, it may be less than ideal for them to make decisions off what would be considered to not be a substantially significant dataset?”
Kinak added that within algo wheels themselves, incorporation of data is not easy for two reasons. One, data is not always consistent across all the vendors that you’re receiving it from, so normalization of data has always been challenging. And then incorporating that data into different tools is not easy.
“As we get better with data utilization, as we get more robust, more granular data, and as machines can process that data faster, and more intelligently, I think algo wheels will become even more sophisticated,” he noted.
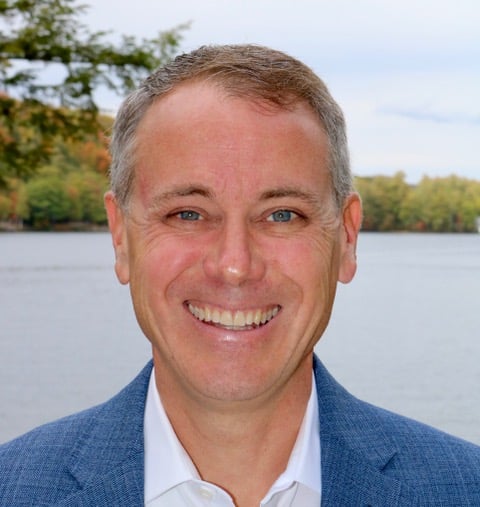
Jeffrey Estella, Principal, Estella LLC, and former Director of Global Equity Trading at MFS Investment Management, said that an algo wheel removes bias, offers automation, provides broad liquidity venue access, and creates a diverse sample size of data to see which brokers, strategies, and venues outperform in certain market conditions.
One benefit of an algo wheel is it removes bias from the broker selection process, he said, adding that when a buy-side trader engages an algo wheel, they are letting the randomization of the wheel and their historical experiences that the wheel has had to establish order routing logic in an unbiased way.
Estella said that most investment managers, big and small, rely on their brokers and outsource much of the execution analysis, including routing analysis, execution slippage, and order type analysis.
The algo wheel is taking that to the next level on the way of automation and creating a large sample size of data to analyze and help determine which brokers outperform under certain conditions and which ones underperform, he said.
“Using algo wheels allows the buy-side trader to focus on the core orders on their trade blotter, where cerebral human input is most beneficial,” he stressed.
Nevertheless, there’s never a one-size-fits-all solution, Estella argued.
“Certain orders on many buy-side desks would be applicable to be traded in an algo wheel, but I’m not stating that it is the right solution for all users or all liquidity needs,” he said.
“You can’t improve what you don’t measure. If a buy-side firm is not measuring how they’re transacting their orders to the micro-routing level, it’s a missed opportunity,” he commented.
Speaking on the risks, Guruju said that volatile market conditions could pose a risk to buy-side desks using wheels: “You need to have kill switches and be able to quickly pivot to a manual process. Without that it could get pretty risky pretty fast.”
Beattie added: “And you’ve got to have controls in place, and governance in place, and a dashboard view of these kill switches.”
Kinak said that he’s not familiar with any of the risks of using algo wheels: “You’re obviously using technology, but technology that’s controlled by you”.
The only advice he would give is that traders need to approach it in a very unbiased manner.
“You have to make sure you don’t have bias in your inputs, because if you put bias in, your results are obviously going to have bias out,” he said.
“Whenever we’re trying to incorporate a new wheel, we’re trying to make sure that we’re designing it in an unbiased way as much as possible, so that the results can then drive our decisions in the future,” he added.
Going forward, Beattie thinks that wheels are going to be more adaptive to event driven information. “As broker wheels evolve, they will move to a more adaptive model that will include both an automated feedback loop of historic Transaction Cost Analysis data, coupled with event driven data emanating from changing market conditions,” he said.
“I look forward to the future of algo wheels. It will be exciting to see the various providers continue to innovate and compete,” Estella concluded.